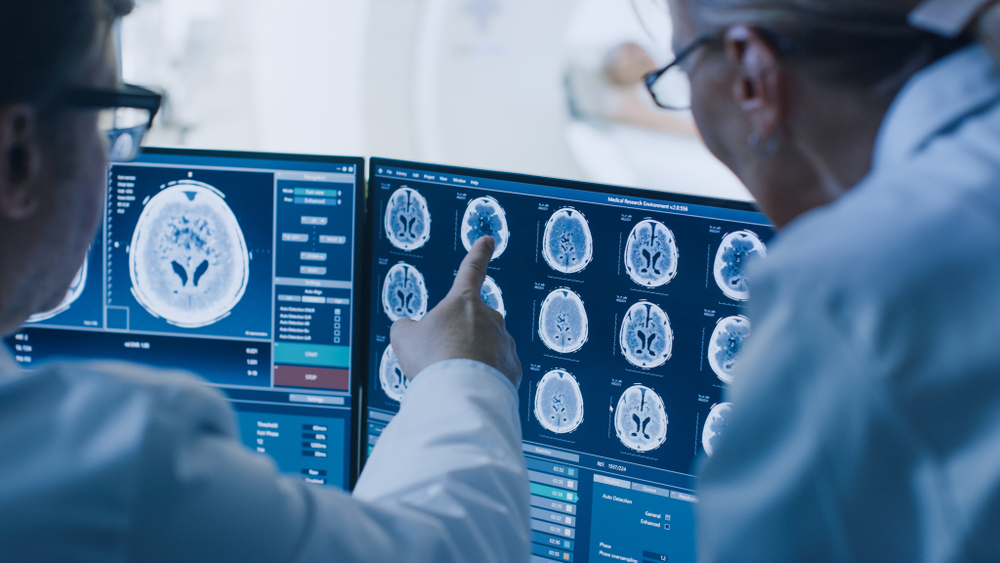
To improve healthcare, there is significant potential to combine labour-intensive manual clinical image analysis with time-efficient automated tools in clinical diagnostics, as well as in surgical and radiotherapy treatment planning. These tools require expert development, drawing on novel techniques from the domains of advanced mathematics and artificial intelligence (AI).
As well as overcoming complex technical challenges, these tools must also earn the trust of the clinicians who would depend on them to provide the best possible care for their patients. But there is currently a large gap between research, emerging technologies and adoption in the clinical practice of automated image analysis tools.
Experts from the Engineering and Physical Sciences Research Council (EPSRC) Liverpool Centre for Mathematics in Healthcare, professors Ke Chen and Francesco Torella, explore that gap and discuss what needs to change to make accurate and ultra-fast automated medical image analysis a reality to improve healthcare for patients and increase efficiency for the NHS.
The view from mathematics research
Ke Chen – professor of mathematical sciences
All models of any nature – whether they be mathematical, statistical or use AI – can work in some cases and fail in others. So, when using a model, it is vital to first understand how robust it is. Within automated image analysis, a number of technical challenges exist including noise, low contrast, irregular geometry and uncertainty.
My research group at the University of Liverpool has developed two new tools for finding regions of interest and combining images that overcome these issues. Working with industry and clinical partners we have developed a novel “selective” segmentation model capable of locating a single organ or feature, blood vessels and stent implants with defined correctness guarantees. An outstanding challenge remains when a feature of interest such as a tumour appears to be identical to normal tissues such as blood vessels or membranes. At this point, clinical knowledge has to be applied, which can result in human error. This is a clear opportunity where modelling could improve the outcome.
We have also developed image registration models that can automatically track changes between an image taken some time ago and a current image, and combine information from images taken using different modalities, such as different types of MRI scans. This work has been conducted in collaboration with many academic and industrial partners including Royal Liverpool and Broadgreen University Hospitals NHS Trust, Liverpool Heart and Chest Hospital, The Clatterbridge Cancer Centre, Cancer Research UK Cambridge Institute, The Walton Centre NHS Foundation Trust, Mirada Medical, and Image Analysis Group.
While it is pleasing to see that almost all mathematics-based models can be developed in AI, currently AI techniques rely far too heavily on annotations, which is the process whereby data from images are labelled. This is much more demanding than the traditional model. AI models tend to provide an incomplete understanding of how predictions are derived and cannot provide the guarantees that are usually expected and required. The fusion of AI methods with traditional mathematical tools is therefore an active and exciting direction forward.
The view from clinical practice
Francesco Torella – vascular surgeon
As a vascular surgeon, over the past two decades I have witnessed tremendous advances in how we diagnose and characterise blood vessel disease. As in other specialties, cross-sectional imaging, such as CT (computed tomography) and MRI (magnetic resonance imaging), has given us the ability to investigate pathology in millimetric detail, aided by sophisticated software that allows complex image manipulation and powerful computers that can handle impressive volumes of data.
The interpretation of images generated by these imaging modalities still depends on careful review by experienced radiologists, who report each scan individually. Some complex scans can take over an hour to analyse, so it is very easy to understand how this mammoth task has become a significant drain on human resources, with substantial financial implications.
Automating this task, by developing self-learning algorithms that can reproduce and even surpass the ability and reliability of an expert radiologist, would be extremely beneficial. This ambitious development is now possible, thanks to advances in machine learning and computational power. I am privileged to have been given the opportunity to contribute to this endeavour at the EPSRC Liverpool Centre for Mathematics in Healthcare because I feel strongly that clinicians should co-design research rather than simply act as end users of technology.
I am very happy with the progress we have made in the past five years, during which we produced and tested accurate AI algorithms to automatically detect aortic stent movement over time – one of the most difficult and time-consuming tasks faced by our vascular radiologists. These positive findings, however, are only the first steps towards an overhaul of practice.
Aside from further fundamental research, new techniques must undergo extensive clinical tests and, perhaps more importantly, will need to gain acceptance among patients and clinicians. Reliance on AI for diagnostics would be such a paradigm shift that political resistance is to be expected, with the safety bar for the new technology set, inevitably, very high. Ultimately, as a doctor and scientist, I hope that future decisions will be objective, and based on accuracy and reliability of techniques that are driven by patient-related outcomes.
Ke Chen and Francesco Torella both work at the EPSRC Liverpool Centre for Mathematics in Healthcare.
Image courtesy of Shutterstock / Gorodenkoff.