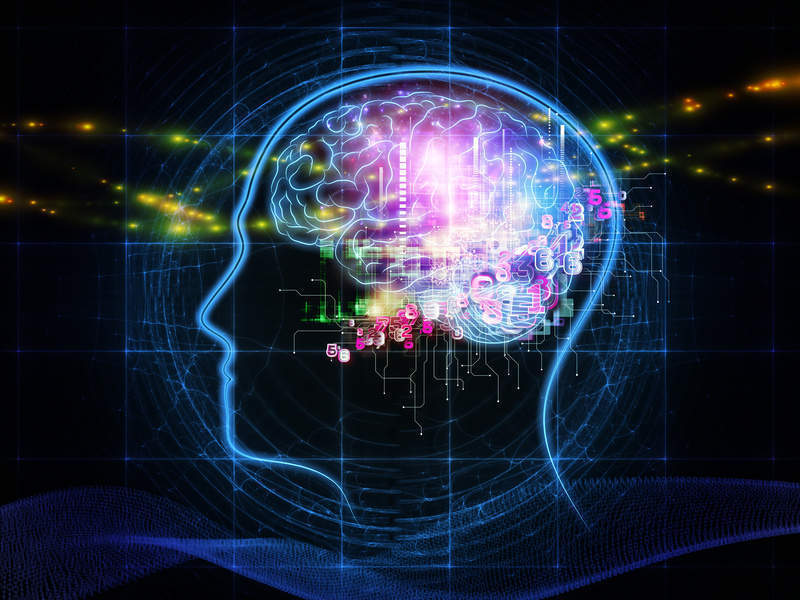
It’s finally time to start planning your survival strategies in the event of a robot apocalypse. For the very first time, engineers have proved artificial synapses can perform a typical human task: image classification.
The engineers, Farnood Merrikh-Bayat, Brian Hoskins, Dmitri Strukov, Mirko Prezioso and Gina Adam, of UC Santa Barbara, and Konstantin Likharev of Stony Brook University, have marked a significant step forward for artificial intelligence by demonstrating the most important operation in a rudimentary artificial neural network (ANN). The engineers’ findings are published in Nature.
Dmitri Strukov, a professor of electrical and computer engineering, tells me, “The particular application we have chosen is not practical by itself, however, more complex and practical applications would just require scaling up the size of the demonstrated circuit.” The network used had about 100 artificial synapses – the making of a very simple artificial nervous system – and with further developments, the circuit may be able to expand its synapses to the scale of a human brain, which has about one quadrillion synaptic connections.
Computer vs. Brain (with added updates). Image: Scientific American
The human brain is still a model of computational power and efficiency for engineers. Yes, the brain constantly signals cognitive biases and logical fallacies, but even the fastest computer in existence doesn’t hold a candle to it. The world’s most powerful supercomputer, Tianhe-2 (MilkyWay-2), computes 15 times faster and holds four times as much data, yet Tianhe-2 (MilkyWay-2) sucks up enough electricity to power about 20,000 homes. The brain is so incredibly efficient that it would require millions of watts fewer to do the exact same job as the supercomputer.
It’s no overstatement to say the human brain is a complex organ. As you read this, your brain is visually processing and interpreting the text I’ve written: millions of neurons in the eye are carrying signals from a certain patch of image, indicating shape/light intensity differences over space (or time, in the case of motion). The signal then gets sent to your brain which derives different levels of meaning through many channels of context – all this happens in as little time it takes you to scan over this print. Change the font, colour or size, and you’re likely to read this and still derive the same meaning.
In the engineers’ demonstration, the circuit implementing the rudimentary ANN successfully classified three letters (“z”, “v” and “n”) by their images, each letter different in style or “noise” saturation. The process is similar to how humans tell the difference between twins, or find their usual items in a £3 meal deal. “While the circuit was very small compared to practical networks, it is big enough to prove the concept of practicality,” said Merrikh-Bayat in the UCSB Current. “And, as more solutions to the technological challenges are proposed the technology will be able to make it to the market sooner,” she adds.
The demonstration was based on 60 memristors (a combination of “memory” and “resistor”), an electronic component whose resistance changes depending on the direction of the flow of the electrical charge. Different to conventional transistors, which reply on a drift and diffusion of electrons and their holes through semiconducting material, memristor operation is based on ionic movement, similar to a biological neural network. “The memory state is stored as a specific concentration profile of defects that can be moved back and forth within the memristor,” said Strukov in the UCSB Current.
Strukov also mentions how the ionic memory mechanism has several advantages over purely electron-based memories, which makes it ideal for ANN implementation: “For example, many different configurations of ionic profiles result in a continuum of memory states and hence analog memory functionality. Ions are also much heavier than electrons and do not tunnel easily, which permits aggressive scaling of memristors without sacrificing analog properties.”
We’ve already established that computers aren’t that cool because they require millions of watts to complete the same function a brain can in just a few watts – so analog memory beats digital memory. If we were to replicate human brain-type functionality using conventional technology, the resulting device would be huge – clogged with multitudes of transistors that require a lot of energy. Now, this isn’t to say more complex ANNs won’t need plenty of memristors to approach the functionality of the human brain – it most certainly would – but it would be much more energy efficient.
There are potential applications that already exist for this memristor-based technology, such as medical imaging, the improvements of navigation systems or searches based on images rather than text. The energy-efficient compact circuitry the engineers are hoping to create would seriously contribute towards the development of high-performance computers and memory storage devices users will continue to seek long after the growth of digital transistors – predicted by Moore’s Law – becomes too unwieldy for conventional electronics.
“The exciting thing is that, unlike more exotic solutions, it is not difficult to imagine this technology integrated into common processing units and giving a serious boost to future computers,” said Prezioso in the UCSB Current.
In the meantime, the engineers will continue to build more complex ANNs with memristor technology, integrating it with conventional semiconductor technology. But could our ambitions to enhance backfire? I ask Strukov if artificial intelligence spelled doom or delight. He replies:
I believe it is rather “delight” at this point. For now we have implemented simple classification (labeling) task which is hardly a sign of high intelligence and I would not worry about doom at this point. The goal of our work was to create efficient hardware and not the development of any sophisticated algorithm for high intelligence. We have considered classification task which humans do well, and developed efficient circuit for implementing it.”
Still, get your survival gear prepared as this might happen soon.