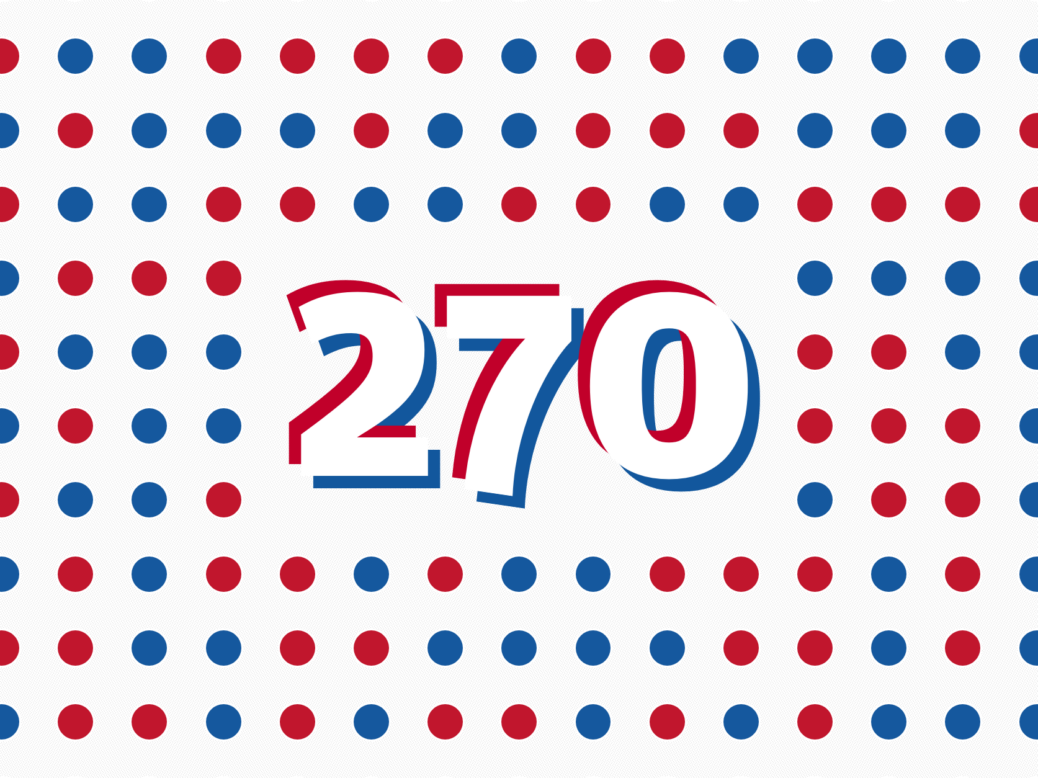
For the first time in its history, the New Statesman is forecasting the outcome of a presidential election in the United States. The model will provide daily projections of vote share estimates and probability outcomes for each US state, seeking to cut through the daily noise of soundbites, tweets and one-off polls to deliver a clear assessment of who is likely to be voted President in November 2020.
With both candidates now officially confirmed and their running mates selected, our model forecasts that the Democrats’ Joe Biden is a clear favourite to take the presidency from Republican Donald Trump, while the chances of an electoral college tie stand at less than 1 per cent.
It needs to be stated that our model is not a definitive single-answer projection of what is and is not going to happen, but a model of probability. A probability model provides a range of results that are all of potential possibility and estimates how likely those results are of happening.
A result with a 10 per cent probability, for example, is not a result that will not happen – it is unlikely, but it is nonetheless still possible. Just because Biden’s chances of winning are better than Trump’s doesn’t mean Trump can’t win. If you had a 29 per cent, or, even, a 10 per cent chance of winning the lottery, you wouldn’t be dismissing your prospects as unobtainable.
How the model works
1. Firstly, we collect national and state-wide polls
We gather voting intention figures, sample size, fieldwork dates, method of collection, and – whenever satisfactory sample size permits – demographic breakdowns.
2. We adjust the polls to account for third parties and undecided voters
Third party candidates are almost always overstated in US opinion polls – Green Party candidate Ralph Nader in 2000 was overstated by as much as four points in the closing days of the campaign. To account for this we readjust those candidates by the typical overstatement seen from previous elections, reapportioning to Trump and Biden equally, with a small share to the pile of undecideds.
The method for reapportioning undecided votes is done in two ways. If the cross-breaks (sub-samples) have an unsatisfactory sample size, we cut and give to Trump and Biden equally. If the demographic make-up is statistically significant, or if there is available data to build an accurate image of undecided voters, we reapportion in a way that would reflect the typical voting pattern of those demographics.
If, for example, all undecided voters are non-college educated whites who have ranked immigration and border security as their number one issue over the course of the past few months, we are likely to recast a significant share of those votes to the Trump column.
This process allows us to make more reliable comparisons between polls by effectively standardising them.
3. We readjust and weight the polls again to account for historic pollster performance
Pollsters often get it wrong and this part of the model process seeks to account for this fact where possible.
This isn’t an exact science because pollsters often change their methodologies over time and cannot necessarily be assumed to possess the same sample-bias or sample-success as four years ago. Nonetheless, it is still valuable to account for those pollsters persistent in their sample and/or methodology bias.
Pollsters persistent in these “house effects” are readjusted to reflect the result of the election. If, in 2016, for example, a pollster persistent in historic bias had Clinton at 52 per cent on polling day (when the actual result was 48 per cent), our model would in future elections readjust that pollster’s Democratic poll share down by four points.
Polls by firms with form for accuracy in previous contests receive greater value in our tracker, and those which haven’t performed as well will naturally receive a lower value.
Pollsters which have performed well nationally but not quite as well in certain states or regions are given values dependent on what it is that they are sampling. If, for example, John Smith Pollsters in 2016 did well at projecting the national share, but not quite as well in Michigan, then that company will receive a high value nationally, but a low one in Michigan.
4. We introduce economic and issues-based indexes
Here we introduce two sets of data which help provide context to the election campaign: an issues index and an economic index.
The economic index is an aggregate of a year’s worth of data which gives a general idea of the direction of the country in economic terms. This tends to shape voter perceptions and enthusiasm about their candidate.
This index combines unemployment figures, consumer spending, and perceived economic wellbeing from opinion polls. When the index is positive (i.e. when unemployment is falling and consumer spending is increasing), the incumbent candidate/party will be projected to do well. When the index is negative, the incumbent will be expected to face a backlash.
Due to the Covid-19 pandemic, the value of a reliance on economic data is less clear than under normal circumstances. It is questionable, for instance, as to whether the metrics we have can provide a concise portrait of the economic situation in the fast changing environment that is a global pandemic.
As a consequence, though it does exist in our model, the value of the index in the weighted tracker is not as big as it would have been.
The issues index, like its economic counterpart, gives additional context on how voters view candidates and their abilities to tackle certain issues – something that isn’t captured fully in headline voting intention questions.
This index is calculated from polls that ask Americans to rank the issues most pertinent to how they’ll vote come November, and comparing them with perceived competence by candidate on each issue. If, for example, racial justice is the number one issue pertinent to how Americans will vote, and if Joe Biden is perceived to be the candidate most effective on that issue, then on the index alone we’d expect Biden to comfortably win the November election.
In practice these indices serve as state-level vote projections, aiding what will become the poll tracker.
5. We produce the final weighted polling averages
The poll tracker works as a day-to-day average of all the recently readjusted polling and relevant index-based data, with more recent polling given a higher weighting.
This section of the process also involves us, metaphorically speaking, “going under the bonnet”. Here we examine individual polls, looking out for consistent headline and demographic variation that in isolation would resemble outliers, but due to their regularity deserve to be accounted for. We use this to create a range of possible values for each candidiate.
If, for example, you have one pollster regularly showing Biden with more support among non-college educated white women than other pollsters, then you have an instance of demographic variation that needs to be accounted for.
Identifying frequent variations allows us to produce a minimum and maximum range of values for both Biden and Trump, accounting for poll error as we go. These ranges are then used in calculating our final state-by-state probabilities, which leads us to the final part of the model process.
6. We simulate the election more than 50,000 times
With our state-by-state probabilities in hand, we take to our simulator. Here we plug in the probability figures, applying our min-max ranges, and introduce variables to make the state probabilities dependent on one another (if Trump wins Pennsylvania, the likelihood of him winning Ohio increases significantly), and from there receive our modal electoral college votes and headline probabilities.
And there we have it – the New Statesman US election model, explained!
With thanks to Josh Rayman and Georges Corbineau for their work in visualising the model and to David Ottewell and Patrick Scott for their work in editing it.