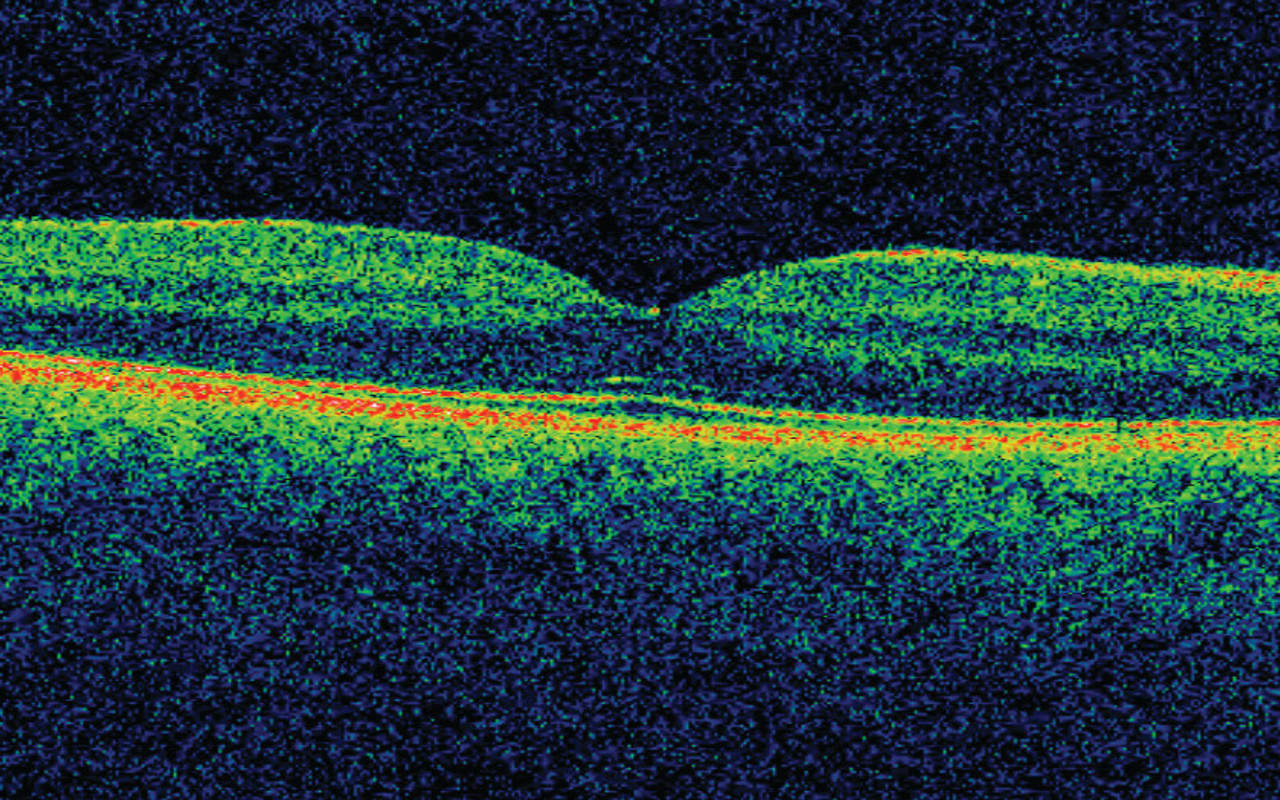
On 15 March last year, the 18-time world champion Go player Lee Sedol conceded a fourth defeat to his opponent, a computer programme called AlphaGo.
To a casual observer, a computer beating a human at a board game is not unusual – the IBM supercomputer Deep Blue beat the world chess champion, Garry Kasparov, almost 20 years ago – but to people who understood the challenge, it came as a surprise. It had not been expected that a computer would beat a human at Go for another decade, and a decade is a very, very long time in modern computing.
The reason Go presented such a challenge was that computing every possibility in a Go game is a task so complex that it is probably unimaginable. For a sense of the sheer dizzying scale of it, try this: a single grain of sand contains, very roughly, 50 million million million atoms.
A game on a 19×19 Go board contains more legal positions than there are atoms in the whole of the observable universe.
Because mastery of Go is not currently possible through brute computational power, AlphaGo won using a different approach. The developers, London-based Deepmind, wrote an algorithm that simulates human learning. The algorithm was then ‘trained’ to mimic expert human players, then ‘practised’ playing altered versions of itself until it was able to play at the highest grandmaster level.
AlphaGo’s victory demonstrated the power of a new kind of computing: artificial intelligence. The significance of AI is that the Deepmind algorithm was not written to play Go – it was trained to play Go. And it could be trained to do other things.
The person in charge of deciding which other things it will do is Deepmind’s Head of Applied AI, Mustafa Suleyman. “We’re inundated with opportunities,” says Suleyman.
“So we prioritise which areas to focus on by finding opportunities to make a very meaningful difference. Not just something that’s incremental, but has the potential to be transformative. We look for the opportunity to have a meaningful social impact, so we want to work on product areas that can deliver sustainable business models, but in equal measure actually make the world a better place.”
This is not the first time Suleyman has spoken to the press about his altruistic aims for AI. In July 2015, Suleyman speculated in an interview with Wired magazine that Deepmind’s technology could have applications in healthcare. Reading that piece was Pearse Keane, an academic ophthalmologist from Moorfields Eye Hospital in London.
“I’m a bit of a tech nerd,” Keane admits, saying that he had been aware of Deepmind’s research for a couple of years. Reading the interview, “when a lightbulb went on in my head, that this should be applied to ophthalmology, and in particular to the type of imaging of the eye that I specialise in, which is called OCT – Optical Coherent Tomography.”
The scanning of patients’ eyes using OCT – three-dimensional scans of the retina that are much better at revealing eye disease than traditional retina photography – is one of the biggest developments in modern ophthalmology.
However, as Keane explains, it is actually a growing impediment to detecting serious eye diseases in the NHS. “Approximately 5-10 per cent of high-street opticians now have OCT scanners in the UK. It’s not like having an MRI scanner – it’s about the size of a desktop computer. They’re usually pretty easy to use and it’s very quick and safe to acquire the scans.
“The problem is that they’ll offer to do the scans, but in many cases they don’t have the training or the experience to interpret them. So what they do is, if there’s any deviation whatsoever from the norm on the scan, they refer the patient urgently into Moorfields or other NHS hospitals to be seen.”
The result, says Keane, is “a huge number of false positive referrals. The people who actually do have sight-threatening disease are then delayed in getting in to be seen, because the system is overflowing.”
This swamping of services could not be happening at a worse time. Ophthalmology is already the second-busiest speciality in the NHS, with more than 9 million outpatient appointments per year.
What’s particularly frightening for Keane, is that among the huge numbers of referrals being produced by the rolling-out of improved scanning are people who have recently developed a disease that will blind them if they are not treated in time.
“Often, someone could potentially have developed severe eye disease, and they could not get an appointment – even if it’s an urgent referral – for weeks, or sometimes longer. If someone’s in a situation where they’ve already lost their sight in one eye, and they’ve started to develop a problem in their other eye – you can imagine, psychologically, what that would be like.”
Keane also points out that this is not happening to an unlucky few, but to a horrifying number of people. “The most important disease, to my mind, is age-related macular degeneration, or AMD, and in particular the more severe form, which is known as ‘wet’ AMD – due to the leakage of fluid at the back of the eye.
Wet AMD is the most common cause of blindness in the UK, Europe and North America. It’s a massive problem. The Macular Society says that nearly 200 people develop the severe, blinding form of AMD every single day in the UK.”
These people need treating quickly. “If we intervene earlier, we have much better outcomes. If it was a family member of mine, I would want them to receive treatment within 48 hours. The national standard for age-related AMD is that they should be seen and treated within two weeks.
“The reality is that across the NHS, that target is not being met, and people are often waiting much, much longer than two weeks to actually receive treatment. The reason for that is that the system is being overwhelmed, and in particular by so many false-positive referrals.”
To make matters even worse, Keane foresaw an even greater inundation of OCT scans. “The big optician chains are talking about rolling out OCT scans across their whole chains – thousands of optometry practices. If there’s no way for us to deal with that, we’re in very, very big trouble.
“It’s as if every GP in the country was given an MRI scanner, but had very limited ability to interpret the scans. Every person who went in with a headache would get an MRI scan, and then they’d have to refer every single patient into the hospital.”
Fortunately, as a follower of Deepmind’s work, Keane knew that the vast amounts of data the OCT scanners were producing were, like moves on a Go board, exactly the kind of thing that can be used to train an AI. “The techniques that Deepmind uses, these deep reinforcement techniques, are successful in the context of large data sets, and Moorfields has probably the largest data sets in the world for many ophthalmic settings.
For just one of our devices, we had about 1.2 million OCT scans.” The AI pioneers were also, helpfully, just around the corner: “DeepMind is based in King’s Cross, and two of the co-founders were UCL alumni – Moorfields is affiliated with the Institute of Ophthalmology at UCL – so I thought I would be a fool not to capitalise on this. I contacted Mustafa through LinkedIn, and he, to my great delight, emailed back within an hour.”
For Suleyman, too, the problem arrived at an opportune moment. “In the last five years, we have made a lot of progress on some of the big milestones in AI. We now have very good speech recognition, very good translation, very good image labelling and image recognition. Many of the things that we try now seem to be working. We have much improved machine learning models, we’ve got access to very large-scale computers, and there’s increasingly enough training data to help us build effective models.”
The millions of OCT scans held by Moorfields presented the ideal dataset for Deepmind to apply its research. “If you think about the number of cases that each of the world’s very best ophthalmologists have seen during their careers,” says Suleyman, “aggregate all those cases in one place and show them to a machine, the machine learning system is going to have the benefit of a much, much wider set of experiences than any single human, or collection of the best humans, could have had during their career.”
While Suleyman says he finds the term ‘artificial intelligence’ unhelpful – “it’s imbued with a lot of anthropomorphic projection, it tends to conjure up the sense that this is a single coherent system that’s doing lots of different things, just like a human” – he is comfortable describing the AI’s interactions with its data as “experiences.”
“In some sense you can think of us replaying all of the scans to our machine learning system in the same way that an expert human might sit in front of their computer and watch scans and case studies over and over again. It’s what we call experience replay.”
Suleyman says the AI also recalls or imagines things in a way that’s analogous to a human mind. “An ophthalmologist doesn’t recall a specific case study that she saw seven years ago. She has an abstracted, conceptual representation of, say, diabetic retinopathy or glaucoma – and that representation is built up through many, many examples of experience and teaching throughout her career.
Those things combine to create a short-form conceptual representation of the idea of the particular diagnosis. We do a very similar thing with our machine learning models – we replay, many times, lots of training instances of positive examples of the pathology that we’re trying to teach the system, and then over time it builds an abstract representation of that pathology and uses that to identify new pathologies when it encounters a new case.”
Just as AlphaGo used human-like judgement to master Go, the system being used with the Moorfields data is ‘imagining’ an abstract form of the disease it looks for, seeing it in its ‘mind’s eye’. “I think seeing it in its mind’s eye is a fair description. It’s generalising from past experiences and making an inference about the new example that it’s seeing at that moment.”
Keane says this is similar to the technology used “to look at photographs on Google Photos or Image Search, or Facebook, to recognise faces in the photos or to be able to recognise that there’s a cat or a dog or a man on a skateboard in the photo.
“The way that the neural networks work is, the raw data from the photograph or the OCT scan – the pixels – are fed into the neural network, and each layer within the network extracts different features from the picture or the OCT scan.
“So, for example, the lower layers of the network will extract very simple features – they might pick out edges, or contrasts between black and white, or other very low-level features – and as you rise up through the network, more abstract features are picked out, so it might recognise that two eyes and a nose indicate a face. And then finally, the output from the network is some type of classification, so it will say that it 99 per cent certain that there’s a dog in the photo, and one per cent sure there’s a wolf in the photo.”
“We train the neural network using a huge amount of examples that have labels; this is called supervised learning. We’re able to give it many thousands of OCTs that have diabetic retinopathy or age-related AMD or other retinal diseases, and then we tweak the parameters of the network so that it can accurately recognise those diseases again.
“We then test the network on a dataset of fresh scans where it doesn’t know the label, and then it will tell us if it classes a scan as having diabetic retinopathy, or AMD.”
Can the system spot eye diseases better than a human? Both Suleyman and Keane say that while it is currently very much a research project, they are optimistic that it will soon be able to ‘grade’ eye scans more effectively – also much more quickly, and more cheaply – than a human.
Keane says he expects people will be able to walk into a high-street optician, have an OCT scan and have it graded by an AI in “two or three years. I don’t think this is more than five years”, while Suleyman says mass adoption is “a reasonable thing to expect over a five-year period.”
Deepmind and Moorfields are not only breaking new ground in technological terms; the advent of AI in healthcare will require new regulation, too. If the eye diseases Keane is hunting were identified by a chemical indicator, it would be subject to approval by the Medicines & Healthcare products Regulatory Agency in the UK, the FDA in the US and others around the world.
And while the use of machine learning is physically non-invasive, the huge reserves of data that the NHS has to offer AI companies are the private property of millions of individuals. It is this data that gives machine learning its formidable power, and the NHS is in a unique position to offer huge, well-labelled datasets; how it is shared, who gets to use it and who gets to profit from it are questions that could fail to be properly answered in the rush to implement this important new technology.
There is no doubt, however, that these questions will need to be answered, because AI is coming to healthcare, soon, and in a very big way. Suleyman predicts that machine learning will become hugely valuable in diagnosing conditions earlier and planning treatment – Deepmind is also working on a separate project that could “massively speed up the process of planning for radiotherapy” – but he says doctors are not the only ones who may find themselves working alongside AI. Managers, too, could be disrupted.
“The hospital environment is such an expensive and complex system. One of the reasons why I think it’s reaching breaking point is that humans are simply overwhelmed by the scale and complexity of managing so many patients who are on so many different pathways, who need so many different tests and interventions. It becomes a massive co-ordination exercise. So, one of the things we’re increasingly thinking about is how we efficiently and speedily prioritise the tasks that get done in different areas of the hospital.”
Few would dispute that the NHS is beginning to creak under its own complexity; AI promises to parse this tangled problem with fast and tireless concentration.
For Keane, this is an opportunity to be seized. “In the next couple of years, we need to work to build on those advantages, because we might have a head start, but that might not be there indefinitely.”