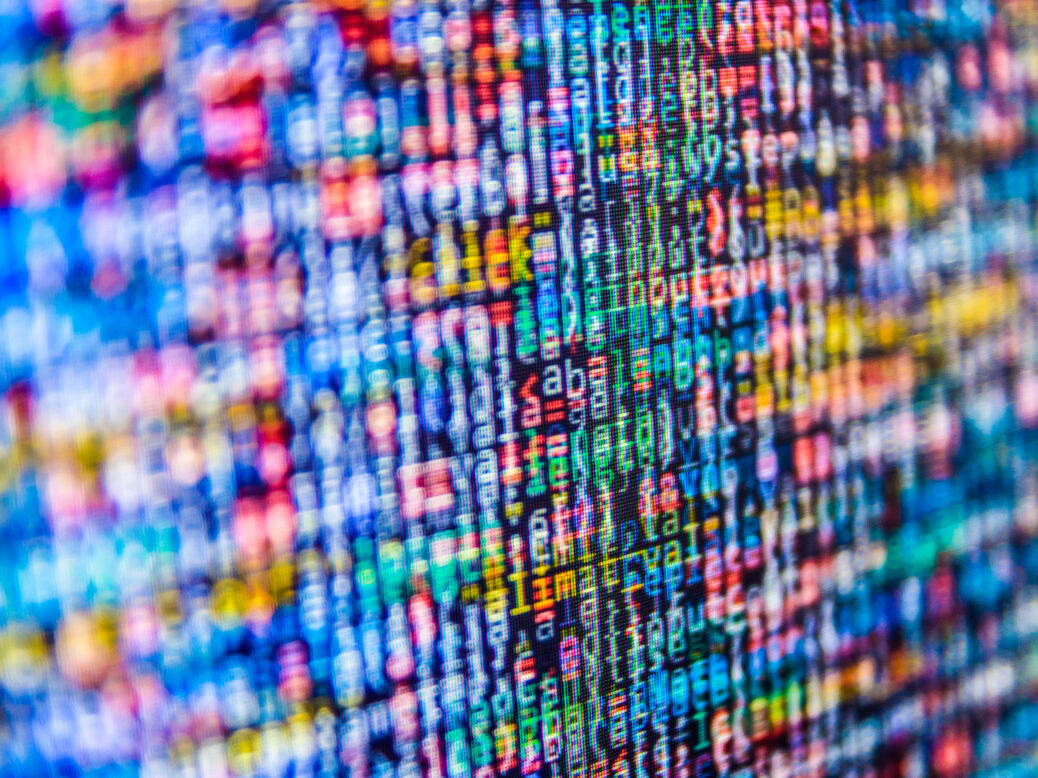
Are public organisations ready for artificial intelligence? Recent tragic accidents involving the Boeing 737 MAX suggest they are not. While modern aviation, including rules-based software it relies on, is overwhelmingly safe, the slippery slope of self-regulation by industry shows the limits of human-machine interactions.
The step towards probabilistic software, such as that used in self-driving vehicles, is not just highly demanding technologically speaking, and uncertain at this point, it also puts public regulators into a uniquely complicated situation. Namely, there are things we may want to regulate – for example, search algorithms that exclude competitors, social media feeds inciting violence – that will change as they are being monitored. The object of regulation is dynamic. And the complexity only increases as we are applying AI to less technological areas, such as health or education, while trying to understand how these complexities affect people, systems and society.
The European Commission has fined Google €8.2bn over two years for abusing its monopoly power in online advertising, shopping and in its Android operating system. This is a highly commendable action. Yet, it is unlikely that will change Google’s behaviour, mainly because such rulings misunderstand the source of Google’s ability to dominate. The market power of big data companies does not rely on their means to pressure websites into using their advertising tools. Instead, power comes from the combination of the sheer endless amount of data about its users and clients, and its code and algorithms that continuously learn about the users and clients.
Code is not only law, but code is also learning. Historically, learning and in particular tacit aspects of if it, such as the ability of teams to work well together, have been fundamental to innovation. Thus, the question is how competition authorities should curtail the ability to learn within big data companies. Fining them for external anticompetitive behaviour forces them to come up with better internal processes – better code – to circumvent not only competitors but also regulators.
Breaking up companies like Facebook, as recently suggested by one of its co-founders, Chris Hughes, would probably not change the underlying dynamics. Radical open source solutions like the data sovereignty approach by cities such as Barcelona is a much more promising alternative.
Put bluntly, AI makes code and algorithms into economic and political agents, and our economic and policy frameworks have limited tools to deal with such non-human actors whose primary goal is to circumvent human agency. This poses the critical question for AI and public policy: what is the purpose of public policy, such as competition policy or seamless public services, and who and how defines this purpose?
In truth, 20th-century public organisations are not supposed to have the capabilities to check, to question, to redefine the purpose of public policy. In this age of super-wicked problems,
the temptation will only increase to cut out the human from the decision-making processes.
AI will be, eventually, taught to learn from millions of cases of purpose, of policy choices – and it will decide. Thus, the question we really need to be asking is not how to create seamless public services, or how to diminish big tech’s market power, but rather who will teach machines about what is innovation and what is political and policy choice?
Rainer Kattel is professor of innovation and public governance at the UCL Institute for Innovation and Public Purpose.